Florida Atlantic University has received a $652,820 grant from the National Science Foundation to establish Florida’s first NSF-funded Major Research Instrumentation Artificial Intelligence and Deep Learning Training and Research Laboratory.
The lab will get major additions to its cutting-edge graphics processing unit cluster. The lab currently includes 18 GPU servers and 72 Nvidia Tesla V-100 GPU cards and a 38.4 Terabyte flash memory server. The GPU cards are among the world’s best technology for artificial intelligence and deep learning. This project will nearly quadruple the number of GPU cards at FAU from 31 to 103 and will increase the onboard GPU memory six times from 381 GB to 2,304 GB.
The laboratory will serve as the training and research platform to support graduate student teaching and research activities across multiple campuses, colleges, and disciplines as well as FAU’s research pillars (FAU Brain Institute, FAU Biomedical Research Institute, I-Heal, FAU Institute for Sensing and Embedded Network Systems Engineering, I-SENSE, and FAU’s Harbor Branch). The lab involves a collaboration of FAU’s College of Engineering and Computer Science, researchers from FAU’s Schmidt College of Medicine and FAU’s Harbor Branch Oceanographic Institute.
“This important National Science Foundation grant will enable us to create an infrastructure for a deep learning platform for health, web services, biomedicine and ocean research as well as other related domains at Florida Atlantic University,” said Stella Batalama, Ph.D., dean of FAU’s College of Engineering and Computer Science. “This laboratory will provide a training hub for our university and industry partners to work closely on advancing artificial intelligence applications to stimulate South Florida’s technical innovations and task force development, which will ultimately benefit economic growth in this region.”
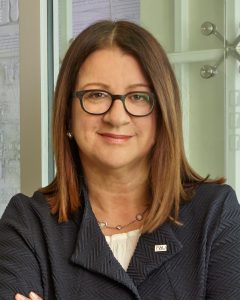
Artificial intelligence and deep learning are making significant strides to transform heavily regulated industries such as financial services, health care, and the life science industry as it relates to tissue engineering, cancer detection, and pervasive sensing. In many cases, training is computationally demanding and takes days or months to have a well-trained deep learning model if carried out on traditional CPU-based systems.